Automating Data Cleanup for AI Innovation with Josh Gray from Artemis
00:00.92
mike_flywheel
What's up everybody. It's Mike we're back here on the pitch please podcast and today I've got Josh from artemus Josh tell us what we're gonna be talking about today.
00:07.20
Josh Gray
Hey, Mike thanks so much for having me on today. You know, yeah happy to dive into you know the the perfect pitch so to speak I'm the founder and Ceo of a company called Artemis and really what we do is we help automate data cleanup for startups. So really what that means is right now. Everyone wants to use these really cool Ai tools and features in their company in in their products as well. Except what they're missing is this key foundation of data quality. Um their datasets aren't ready. You know that old idea of garbage in garbage out. Our platform is here to help solve that issue and make sure that we can scale that job to be done which is cleaning data which is super tedious and technical and how to make that as simple as possible so that anyone in an organization can also clean data on the fly and then use these amazing downstream Ai tools to really you know their full potential. Um, so that's really what we're trying to do here.
00:59.36
mike_flywheel
That super topical and I don't think we're going to be getting ah too far without asking a ton of questions so much to understand about this ai space and people are definitely going to want to check out the balance balance of this episode because I think if you're even considering diving into Ai or you're an existing company and I talked a ton of them. Trying to figure out where ai fits within their business strategy data obviously is a key component to that so happy to hear that you're solving this problem and can't wait to learn more but before we do learn more about Artemis. It's important to know a little bit more about you.
01:21.60
Josh Gray
And.
01:31.32
mike_flywheel
Um, give us a little bit of background like where did you start? What got you to where you are now working at Artemis and how long has artemus sort of been been building.
01:32.60
Josh Gray
Um, okay.
01:39.30
Josh Gray
Yeah, totally so. My background's in finance I'm not ah I'm not a technical person by trade. Um, you know it's why I have a co-founder who who's an amazing engineer. So starting finance I worked in the actually ah the alternative data space. So I'm not sure if you're too familiar with it. But. Gets creepy really quick but essentially how do you use crazy wacky datasets to help inform you know, financial movements and markets and so you know the famous case study. There is you know hedge funds in the early two thousand s would get satellite images of you know Walmart or Home Depot parking lots count the amount of cars coming in and out and they could within some degree of ah. You know relevance to calculate. How much revenue they were going to have and if they were going to miss beat or beat earnings that quarter so did a bit of work in that space. It was really cool and what I learned really quickly was I was on the financial sentiment analysis side so understanding. You know how does you know How does you know.
02:20.97
mike_flywheel
That's crazy. So.
02:32.54
Josh Gray
Tesla shareholders react. How does the soft react on good news versus bad news. But what was really cool as it um was I learned exactly where value accrued and in data and in datasets specifically because you know project I was on. We'd sell these two you know hedge funds or or people and so where we found that really where I saw accru was in its quality. Ah, dataset had to be you know purified. You almost think of it almost like water in a way you know it had to have a level of purification where you had multiple pieces of data and multiple datasets flowing into one you know master table. Um, at the end of it need to be super crystal clear and so that was kind of what started me thinking about this problem. Now. Fast forward flash forward a bit I actually started my own company. My first startup back in 20 right? when the pandemic happened I started a local business with another cofounder. The idea was stay home support local. It was 10 local products in a box ship your home. Um, and it was before curbs I'd pick of it actually blew up. We grew to about a million dollars in revenue um and it was acquired back in 2020 so um, during that experience scaling a company I was like why don't we use data to understand where our best customers are coming from understand what's happening in our business and I found as a nonengineer. It's actually really hard. To get that gold. You know that gold seal quality level that I was looking for. You know it was a lot of manual work. A lot of tediousness and you know as a finance guy I love excel but I'd rather I spend you know 5 hours a day in it and so that's really you know what got me thinking. Okay well what tools are out there and when I saw the market I saw that there wasn't really this piece.
04:05.36
Josh Gray
For nonizing little people um to be able to really dive into you know this problem of of quality and so um, that's really what got our gears thinking I actually reconnected with um, an old friend of mine from middle school James Willies my cto he was just finishing up mechatroics at Waterloo had a ton of internships and experience doing this work. Um, and he felt this pain point as well. So we teamed up about a year and a half ago and I've been building ever since.
04:27.74
mike_flywheel
Yeah, that's really cool now when you started that first startup did you do that while you're still working in the financial services industry. Did you always know you were going to be an entrepreneur like how did that that that that was a big change. Oh wow.
04:35.45
Josh Gray
Yeah, so was actually no so yeah, totally so that was actually while I was still in University so I did that and I actually sold the company the day after I wrote my last final exam so I was pretty crazy. Yeah, it was pretty awesome. So um.
04:48.40
mike_flywheel
Yeah, that's quick as.
04:53.63
Josh Gray
Totally fell into it. It was one of those things where covid it was literally like covid I think the and Nba shut down on the Wednesday like Rudigo Bear got covid like the Nba shut down and that was when the world was like holy crap. This thing is real and then it was like we launched the website that Friday like it was like you know what we're going to be home for two weeks I had a summer job lined up that I was going to go work at. Um, that ended up getting canceled because of covid and so I was like you know what? let's do it in two weeks turned into two months turned into 2 years and you know a lot a lot of me finish school debt free which is pretty awesome.
05:17.13
mike_flywheel
Wow, That's that's a really badass story and I guess the interesting thing is so you know some things got canceled. You started working on this but before that like. You You were in University you were working in financial services learning some stuff were you planning to graduate into entrepreneurship or did you think you were maybe going to go work in that corporate career. Oh wow.
05:37.17
Josh Gray
No, no so I actually had a job lined up on Bay Street I was going to go work in the Toronto but and ah and like in toron on the finance and the finance sector. Um, and you know I was just about I was just about to sell my company. And this idea in the back in my head of you know I want to start a company that helps teams clean data. Um, and so I actually finished my studies a bit early a semester early and I went to coleton university and they were just launching an innovation hub and so they had this program that said we'll pay $10000 and we'll give you a class credit if you want to start a company. And so I was like you know what might as well take advantage of that I did um and launched artemus at that time and by the time I was ready to leave school I was you know artemus was kind of up and rolling. So.
06:23.83
mike_flywheel
That's that's super interesting and so do you think that the that the success of your first exit primed you to be like let's keep going down this train. That's when you found that program. Is it.
06:35.50
Josh Gray
Yeah, totally totally I think I think for me personally it was I knew that there would be jobs there for me at the end of the day. Um, and that's you know what wanted you know I wanted to get into this. Um I think as well I was very fortunate to have an exit.
06:43.73
mike_flywheel
Um, yeah, and.
06:52.64
Josh Gray
Thinking Canada um, you know it can be you know sometimes you have to have quite a bit of money you know to get a company started. You know we just started paying ourselves recently. So it was a long time for I started collecting a paychecky and so that exit really helped me you know invest money to the company. Get off the ground hire for a few engineers pay ourselves and you know survive for the first year and a half and so um, yeah, that really helped me on so I was able to kind of say you know what worst case this doesn't work out in a year I can always go get a job but I was in a very fortunate position to do that. Oh yeah.
07:21.30
mike_flywheel
Got it now you know I want to talk about the timing of this because when you had graduated school exit Congrats on that by the way that's that's huge and to your point not not every Canadian company gets the opportunity to do that Big exit small exit when you get to sell a company. It's It's a big milestone.
07:34.40
Josh Gray
Um, thank you.
07:40.60
Josh Gray
It's a process. It's a process I mean it's ah hopefully maybe I'll do it again someday. But it's ah it it keeps you up on that I'll tell you that much. Oh it exactly.
07:41.33
mike_flywheel
Um.
07:47.93
mike_flywheel
Yeah, it's not. It's not like a one day transaction. It's not like going to your grocery store. There's a little bit of legals involved and back and forth and book scrubbing. Um, but like you, you'd worked with data and obviously now you're working with data. But.
07:58.17
Josh Gray
Then.
08:02.80
mike_flywheel
There's 2 elements that I'm like fascinated by did you naturally know that this is what you were going to start next or was there like an ideation brainstorm we like hey we're kind of done. Let's have a breather by the way like I kind of want to start something new. Let's tinker I've got things that I'm good at I've got things that I know and then you got here i.
08:15.59
Josh Gray
Yeah, yeah, so I knew I wanted to solve the data problem I Knew that was the problem I was going after the problem. The problem was crystal clear and I talked to other people about it I talked to a lot of other founders I talked to people in the street and that was like so clear which was.
08:22.40
mike_flywheel
Okay, like that was in the back of your head this whole time.
08:34.65
Josh Gray
Getting this quality is very very difficult. Um you know and so when I knew that I needed to upskill myself in terms of the industry. So I actually spend probably 3 four months with my head and books on websites on platforms learning understanding. What the heck is a data warehouse. What does etl mean right? What is you know you know all this sort of stuff what is augmented analytics right? What is search-based analytic analytics all these terms and ideas and terminology I need to understand now that's paid dividends for me. You know we joke that a lot you know a mut.
08:51.47
mike_flywheel
Yeah, yeah.
09:06.94
Josh Gray
Investors or clients or partners they think I'm technical and I have no idea how to actually build any of this stuff I understand what it means but I really had to take some time to upskillle myself and learn what all this stuff actually means um because there's a lot of information you got to know? Um, obviously I'm still learning every day. There's so much I don't know. Um.
09:11.12
mike_flywheel
And.
09:25.51
Josh Gray
But I really spent probably the first 3 4 months just educating myself on the industry 2022 yeah yeah
09:27.64
mike_flywheel
And this would have been like like 2021 Twenty Twenty Two got it. So in 2022, that's when you'd started this which I'm like kind of correlating with when like Ai got really hot which it wasn't at the start of 2022 like you were a little bit ahead of this. It feels like you just want to solve a data problem and then you're like holy shit Ai's on fire.
09:40.20
Josh Gray
Um, yeah no no yeah I mean I was well I think we we? Yeah yeah, exactly we really lucked into it which was this was a problem this been. This has been a problem for 2030 years the issue though is the end user. It was never was kind of sidelined right? You know there's a joke. Actually it was funny I was just starting somebody button this yesterday. There's a joke in the industry that you know data equality is one of those things that everyone thinks important is important but nobody takes responsibility for um and so what ended up when ah, what ended up happening was. Kind of. We're going to market. We were understanding this pain point and people wanted to fix it but it wasn't really a priority because the end user didn't actually have a way to interact with that data anyways, right? The flow was the end user would ask a question to the data team hey I want to know about our burn. Let's say right? I want to learn about x.
10:27.97
mike_flywheel
Amen.
10:34.93
Josh Gray
Um, the data team would go find the right pieces of data clean it prep it you know massage it in the way they want build a dashboard send them the dashboard get feedback right? And there's this constant loop. But what we saw with Ai was it completely changed the dynamic which was end users now have a way to ask whatever question they want. But what they need and what they're lacking is that clean data set and so it then kind of re changed the dynamic it changed the dynamic so to speak which was it no longer was on the on the analyst schedule of let me just get you the dashboard. It's no I have to clean this data set aapp because my because my end user needs it to ask the right question they have um. And people then started to realize realize okay crap. Our data quality is not where it needs to be and that's really kind of what got the flywheel spinning from people understanding how painful this actually was so yeah, you're right in terms of you're kind of going at this problem I'd say about like eight months before chat gbt really took off. Um, yeah, so.
11:28.70
mike_flywheel
Um, yeah, and to be fair like if you're listening to this like Ai wasn't invented with Chad Gpt like it's been around for a while. So there's been a data problem for a while. There's been Ai needs data warehousing needs. Well before but I think the democratization in the.
11:36.10
Josh Gray
Um, oh yeah, totally yeah yeah.
11:45.60
mike_flywheel
Boom and the speed where people are like I don't want to miss this training and I need to figure it out. That's what kind of clipped. So.
11:46.98
Josh Gray
Run. Well yeah, and also yeah, and and you have you have you know end users and in the way what I mean by end users. That's probably define that is you know people in the business every day you know you have frontline people product managers you have you know, financial analysts all these. You know sorts of roles of the companies who need insights at their fingertips. It's almost more frustrating for them because they can actually pass the question themselves right before they couldn't do that they had to ask the analyst to build it all for them whereas now they're saying hey I want to ask where what our revenues at what our burns at but where's the data set. Um. And so that that boom sort of speak really kind of you know? yeah flip that dynamic on its head and has made you know this part of the process way more relevant today. But yeah, you're absolutely right in terms of it's been relevant for 20 years
12:29.68
mike_flywheel
I got it in root. Did you see that inflection because I assume at the beginning of this like you had a product. There's probably Mvp and then you start rolling out with customers but Eight or nine months and did you see an inflection in demand when people were trying to.
12:37.55
Josh Gray
Um, yeah, yeah.
12:43.57
Josh Gray
Yeah, well totally totally what we saw really was this gap between knowledge within an organization and what these Lm tools could provide and what I mean by that is you had this almost like euphoric idea of oh.
12:46.38
mike_flywheel
Like they were more proactively trying to solve this at that point and.
13:01.86
Josh Gray
Chat qute is going and answer everything I have but then we learned you know probably six months or a year later that you actually there's a big context got missing and there's been studies done by Google Dmin that have shown that allens are not actually that great at jumping context. It's really good on what it's trained on but not necessarily new stuff. Um, and so. You had all these people you know we built like a rough you know, chat with your data prototype just to see what people would do and again they would go to use it but they'd be like oh my revenue tables aren't aligned so how do I do that and you're like well you got to figure out how to do that first and then you can ask the question and so yeah, we definitely saw that. Um, that disconnect especially within kind of where what Ai tools were missing. Yeah.
13:41.88
mike_flywheel
So maybe let's talk about who this is for um, you know it's a data cleansing tool is this for like individual consumers it for businesses startups large enterprises who's like sort of your primary audience today and maybe that'll evolve over time. For example.
13:49.14
Josh Gray
Yeah, yeah, yeah, it's definitely going to evolve right now we are definitely moreed tailored sorry towards a more technical role. The reason is because right now the role of cleaning data is extremely technical and really trapped within data teams. Um, we eventually you know our mission is to scale that function outside the data team and across an organization. But there's a whole bunch of ownership things and things like issues around that. So right now. Really what our product supports for data teams specifically more technical data analysts. Um, you know they might know Sql They might know a bit of python. Um, we help automate and really speed up that process to clean these data sets at scale now in terms of company size. We're a pretty horizontal tool. We sit on top of what's called a data warehouse and so therefore we really work with all types of data. But everywhere really I'd say you know we work with a few large enterprises but mostly in that kind of series a post size because you know once you get a you sit and you kind of hit an inflection point where once you have enough data kind of becomes hard to manage moving forward.
14:54.96
mike_flywheel
So yeah, let's maybe talk about that because I think there's like that some people don't know that there's a world of data Beyond like the number of columns and rows in an excel table. Um like what is like the amount of data where people start to like think about a data warehouse. Um, and.
15:04.59
Josh Gray
Um, yeah, yeah.
15:13.75
mike_flywheel
Where does that fit in because you know I'm you the average company collecting tons of data but it's definitely in different ways. It might be stored in tons of excels to be honest or online for like where is that like inflection point and where does a data warehouse fit in as as a primary localc point to the.
15:17.50
Josh Gray
Um, yeah, yeah.
15:28.53
Josh Gray
What one of the ways we really see it is when you start to see saas for all or tooling start to really grow across teams so that comes typically around employee 10 plus all up to employee 50 What I mean by that is. Hit a scaled within a company that you know maybe you're using salesfors as a crre as an example, um, but then you're also doing a ton of customer with slack. Well those aren't actually integrated so when you ask you know Salesforce Ai you know what are our customer issues right now that we're hearing. It's not only going to catch a portion of it and so once you kind of start to see that that. Bifurcation I guess and that's split between a bunch of different saas tools. That's where you start to see teams wanting to centralize it all so they can actually start to get a more holistic picture again going back to that context if you don't have all the context you know you're not going to get the right answer. Good example of that is you know we see all the time startups will come in and you know. They charge. You know they customers via stripe but they do all their refunds and and you know wholesale ordering through Quickbooks. Well, you're not going to be able to calculate revenue through stripe you have to combine those 2 sounds simple which is 2 sources but you can see how you know that exponentially scales. You know, moving forward. So that's typically I'd say when we see it. Smaller companies though. They can do a lot of this stuff via spreadsheets and quite honestly if you're that small the company you know 5 10 where you know where we are. You should be doing that you know right? You should actually have a really nity gritty hands-on view of what your data is actually saying.
16:54.93
mike_flywheel
So ah, we 2 things 1 a shut out Microsoft if you do do those things in dynamics 3 six five and teams there is context but that still doesn't solve the data problem. You still have data from so many things and at some point you still take the data from those.
16:56.98
Josh Gray
Um, yeah, yeah, yeah, yeah, totally.
17:09.55
mike_flywheel
And to reason over it more broadly for other things. There's still this tool needed but just given my shadow you know since it worked there a little bit of integration. But what I wanted to talk about was like maybe there's a profound number of customers actually because you you know we're kind of talking in terms of employees but is there like a customer volume that's sort of like the trigger point for people to be like.
17:12.52
Josh Gray
Tory Love it.
17:19.89
Josh Gray
Are.
17:29.13
mike_flywheel
Hey I'm sitting on enough customers that there's probably enough data here that I could start to make something real out of it or is that not really how you think about like where you fit in. So.
17:32.91
Josh Gray
Um, a.
17:37.40
Josh Gray
Yeah, we haven't we haven't measured that ourselves. But I think you're definitely right in terms of we've been collecting just data without knowing what to do with it. Um, for so long and there's definitely a point where when you start having enough customers calling the.
17:44.81
mike_flywheel
Yeah, yeah.
17:52.83
Josh Gray
On your platform you want to be tracking and understanding. What's happening I wouldn't know what that specific number is but I mean I'm sure you know there's definitely validity there.
18:01.48
mike_flywheel
It's I guess more along the point of what are you trying to do. So once you start to try to reason over it and whenever you're trying to bring it together from multiple systems regardless of what they're that's kind of where the breakpoints are first.
18:05.10
Josh Gray
Um, yeah, yeah, yeah, that's what we've seen today exactly.
18:16.20
mike_flywheel
And got it so where um, where does Artemis specifically sit or what does it do and maybe we just break it down. Obviously we don't need visuals to use but maybe like let's talk about generally how data is stored and then where and how some of those problems exist.
18:23.51
Josh Gray
Um, yeah, yeah, yeah.
18:32.55
mike_flywheel
And then that way. Ah we can start to figure out like where Artemis plugs in or overtop or how that kind of works and.
18:34.97
Josh Gray
Totally yeah so I'll kind of explain like the typical you know data flow what you think of as like the modern data stack. So typically in the modern data stack. What you have is you know you kind of picture this from left to right? you have you know data sources. So that's going to be maybe your own application. Maybe it's saas applications that you use you know. Dynamics 3 65 maybe it's teams. Maybe it's you know hubspot maybe it's stripe. Maybe it's ah you know Adobe Analytics I'm not sure. Um, but typically you have the data trapped within these saas tools then what you want to do is use. What's called an etl tool or data movement tool. Let's think airbyte or fivetran and they're going to help you take. You know they have pre-built integrations that help you take data from these sources into a centralized location now that centralized location is going to be what's called a data warehouse that can be set up. You know on snowflake that can set up on databricks if you're really data heavy. It can be set up on maybe Amazon reds shift google bigquery um. Or you know we see a lot of postgres databases as well kind of using that in that area. So that's kind of like the first part of you know what? you call this state of stack now if you have custom sources. You need to build those you know obviously custom you have like a lot of scripts and that can be very time consuming but that's really kind of part one or I say the first half which is how do you move? Data. From the outside and into your organization. So then once you have your data in your data warehouse you then want to work with it in some way through a visualization tool and so this kind of is part 2 of the process with a second half which is you have to take data in table form or whatever format. You have.
20:10.25
Josh Gray
Clean It maybe merge it together through maybe let's say a transformation tool that's where we would be and then you send those finalized, Beautiful clean tables into a visualization tool like power bi like looker like Tableau toward visualize it for you know end users that's more of that Dashboard Tool. So. Does that make sense kind of from end to end.
20:27.79
mike_flywheel
Yeah, yeah, and let's can we make it. Let's let's make it real through like something people will like generally know like contact information. It's like a contact name email address address phone number and so obviously that exists within all these systems right? So you know hey.
20:34.50
Josh Gray
Yeah, yeah.
20:45.59
Josh Gray
Yep.
20:46.84
mike_flywheel
Ah, Josh and Mike both exist in my crm. They both exist in stripe they both exist in. Um, you know my web portal and maybe in some like maybe it's a patient record or something. Um, what do you mean? I can't just put them together isn't it always? Mike.
20:49.21
Josh Gray
Yeah.
20:57.10
Josh Gray
Exactly? Yeah yeah.
21:02.66
mike_flywheel
Tibito and and Josh and we've always got like an email address and a phone number like where is the the dirtiness in that data when you try to bring it together. So.
21:07.76
Josh Gray
Totally the dirtiness ist typically honestly unfortunately at the it's human error. It's you know in in your crm we might have the right name but in the crm let's say you put my company as Artemis right? whereas in you know, let's say ah. Billing tool like Quickbooks or stripe you put it as our legal name right? R missing and code software right? there. There's a difference and if you ask the question. It's not going to know data is very very fickle so you have to be very you know strict with it so to speak right? and then you miss you have a patient record where you have an a you have an address from three years ago well I've moved few times since then so it's actually out of date so you actually don't have the right address or you have 2 conflicting ones. Um, so those little things seem like little annoyances but when you get to the scale of hundreds of users thousands of users millions of users. You're not going to go through it. You know like a spreadsheet but 1 by 1 to clean it all up. It's just going to take too much time. And so that's really where the dirtiness starts to get to then? You also have a difference in how those Yeah yeah, yeah, yeah.
22:06.74
mike_flywheel
So it's like the the initial like rectification of the records like you're like Matt hey match lookup for duplicates or things that are similar merge and then start to make a choice of do you take the name from this one the address from this one. The phone number from this one because they're sort of.
22:24.39
Josh Gray
Yeah, yeah, yeah, except it then goes a letter deeper which is well. It's not like all these tools you can just copy and paste into an excel spreadsheet and they all have the same columns and things like that right? you're going to have your crm which might say email underscore contact.
22:24.60
mike_flywheel
Close enough merge and then delete these ones and this is the new format is that kind of the concept that.
22:42.71
Josh Gray
And then you go into your billing platform and it's going to say email underscore contact. Underscore June let's say for as of last month well right there you have to actually differentiate that maybe if you're writing sql or in python or you're downloading them. They're actually differentiate and. Let's say that you you know brought on you know your billing platform in August but you brought on your crn in March you have to make sure that those dates line up. So there's all these different vectors and ways that this that these datasets are really not meant to work with 1 another um and the challenge is how do you make them work together and how do you make sure that it's accurate. Because the worst you know the worst thing that you see in data and it happens all the time is you go to a board meeting. You have a nice you know you've been working all you know all week as an analyst cleaning this data set you go to a board meeting and you say all right here's revenue for q 2 banner year we're having a great one. It's 15000001 of the directors looks at it and goes. There's no way it's 15000000 because we did 4000000 last year and there's no way we've you know, quadrupled or whatever our our revenue? Oh well. Actually what we did was by accidentally we we double counted something right? You start to lose faith in what those numbers actually represent and therefore you start to lose faith in in the whole data processs altogether and. And so it starts to get again. Yeah as you can see messy really quick. Um, so yeah, yeah, so right now it's been a very technical job. It's engineers and data analysts who go in and write code and have very you know we like to say deterministic ideas of.
23:58.33
mike_flywheel
So how do you fix that.
24:12.38
Josh Gray
Have xtable and ytable and you're going to join them based on these very specific parameters. Um, how we see it. Environments is a bit of a different way to do it and because what we want to be doing is making sure that you can be building across all your data sac not just or all your data tools and all your data tables so to speak. Um, so at ours we you know we sit on that transformation layer which is pulling data from a centralized source like a data warehouse and cleaning it in our platform so that it's ready for a visualization tool to take advantage or downstream ai tool. Let's say and and so we we have 3 kind of layers to our platform. The first is the data layer. We then have the logic layer on top of that and then the last one is the automation layer now the data layer. Um I'll kind of get into each piece the data layer what we do is we give structure so we understand and we have we have our own um contextual ai that we've built out that gives structure to how an organization's data is formatted so we'll understand. Hey know what this payment stable from stripe and this payment table from quickbooks they're actually pretty similar so you know what we're gonna we're gonna give them a nice tight relationship and we kind of neighborhood. How data fits in with one another and that is actually allows us to downstream make a much better experience for our users and so. Once we you know, give structure or kind of you know, think of it almost like a map right? We're building a Google maps you know idea of how your data is structured and set up. We can then understand through logic but through our logic piece of the platform. How do you actually think of this structure. How do you actually think of how your data is formatted.
25:41.30
Josh Gray
You know, let's say with you know with a startup they they define revenue completely different than another startup right? If you're a subscription business versus you know, let's say ah a pay up from once type of business revenue is going to be different and how you calculate that so each business needs to have the ability to say all right here's how we define revenue here's how we define burn here's how we define product you know user churn. And they need to be able to do it and so we give them the tools to do that through our logic layer. Um, you know some of those low-code no code. But the idea being that they can easily say all right here's how I want to massage this data in this way and then the last layer is that automation piece and that's really where the fun Ai stuff comes in which is you know, really cool these days which is. How do you take those logic pieces and actually automate those workflows so that analysts can you know see how structured clean it in the right way and then automate so they can move on to bigger and better things. Bigger fresh to fry that's kind of how we see the platform stacking up today. Yeah.
26:28.89
mike_flywheel
Got it so and it's really like a human assistant right? because that logic layer you know you're suggesting but at the end of the day you're defining the the actual thing and then you're you're then deciding to automate it and alleviate that time. So there's human.
26:39.47
Josh Gray
Yeah, oh yeah, um, absolutely.
26:46.65
mike_flywheel
Humans along this but the idea is you're assisting them in something that would be otherwise super manual as a process and take way more time and.
26:50.81
Josh Gray
Yeah, totally and you know we we see that we're able to take you know an analyst time from you know, maybe 2 3 days to build a model and you know an end-to-time workflow down to you know an hour or tw hour so there's a huge amount of time saving and you also you know, maybe you don't know how to write in python or sql. Um, you know you don't have those technical chops but you understand how the flow works you can still build and own platform.
26:58.26
mike_flywheel
Wow.
27:10.26
Josh Gray
Um, because we're able to automate a lot of that work for you. But absolutely no, there's um, there's always a human element. We really see ourselves as a tool to empower these analysts because you know everyone's being haulen the end of the data analysts. They're all dead. They're you know they're not going to. You know they're not going to make it well if anything we've seen higher and higher quests for throughput. Through these through these you know, incredible people and so they need to be equipped with the right tools because you need to have that human element to to really understand what's happening.
27:29.47
mike_flywheel
10 Tons tons.
27:38.59
mike_flywheel
So um, tell me about like where this sits do I install it in my data warehouse on my cloud of choice is it. Do I have to import everything into Artemis or do it sit in reason over my data within my data warehouse for speed and processing how does that sort of installation work.
27:45.91
Josh Gray
Um, yeah, yeah, yeah, yeah.
27:56.20
mike_flywheel
You will.
27:57.20
Josh Gray
Yeah, So we we try to make it as simple as possible. So we sit on top of your data warehouse which really means that we don't actually pull data out what we do is we pull out Metadata So in a way What we can kind of do is we pull up Metadata and it helps us understand you know column names and table names things like that we can then help you build the right logic and then once you've kind of saved a workflow. It actually goes back to your data warehouse and. Structure warehouse to do whatever we told it to and so what that means is from a security standpoint we can ensure data staying in the same Place. We're not pulling it Out. We're not changing servers. You know you can have that piece of mind. Um, you know we can never go in and understand what's actually going in with the new data because we just see the metadata layer. Um, so that's where we sit on top of and it's super easy to get on boarded our engineers have done a really good job of that.
28:40.91
mike_flywheel
And that's sick. Um, so who's who's sort of using this today and you know like what are some of the ways in which you've quantified I know you talked about it a little bit from like days to hours for analysts. But what are some of the initial you know impacts that you're seeing is it companies trying to.
28:48.24
Josh Gray
Um, yeah.
28:56.97
Josh Gray
Um, yeah.
28:58.44
mike_flywheel
Use this for internal reporting is it hey we're trying to use this so that we can unlock our data and create new revenue models and business models like where are you seeing this fit in and who is who's sort of using Artemis today. So.
29:09.51
Josh Gray
Yeah I think bit of both I think I want to want to clarify that I think there's this whole idea you know you hear of dark data all the time right? All dark data all this sort of stuff you know, 60% of data is never used now. The thing is If. Of the structured data out there. You know which is entirely of format. There's so much information that we still have been able to extract and so for now a lot of the use case is automating current workflows which is you know, maybe pulling in data from a warehouse making sure it's clean and you know reported for financial Metrics. So We see a lot of internal reporting. Also are starting to see a lot of experimental reporting and what I mean by that is you know going in and diving down you know, kind of more data scientist idea of how do you dive down. Ah a train of thought and go really into the Nitty-g gritty of what your users are doing in your product. You start to see those pieces as well. Um, so I'd say those are kind of the main too of of really exploration but also automating those internal workflows that they they've had up for a while but it's still a very manual and tedious process.
30:06.41
mike_flywheel
I got it. Okay now Define Dark data for me I mean not everyone listening in knows what that means. So I I feel like it's worth at least talking about this dark data concept.
30:09.91
Josh Gray
Ah, yeah, yeah, so I'll kind of go back to another definition then and again I apologize there's so many to out. There's so much terminology like I said it took me months to learn it. Um, so you have.
30:22.60
mike_flywheel
This is why we do this though people are learning right? Yeah, so.
30:28.78
Josh Gray
You know, kind of 2 buckets structured and unstructured data now structured data is kind of what you think about when you look at excel right? tables and rows columns. You know, columns and rows tables of of data. You know you think of a database you know, very you know rigid and structured so to speak now on the unstructured side. That's where you have more images and videos and maybe it's text and a lot of that's becoming much more accessible thanks to Ai and Multimordal Ai and that's really really cool now the way it's split up though is but 80% of the world's data is unstructured right? You can think of the internet blog format videos on Youtube all that sort of fun stuff. Um, so not a lot of it is actually in a structured ti format. That's easy for ah you know, ah you know someone to work on or or work with and so going into an organization those weights obviously different by organization or maybe thirty seventy maybe 4060 but they stay somewhat similar which is. Majority of data you think of you know what you have in you know, sharepoint or what you have in teams. That's all unstructured because you know there isn't you know a table a tablet format to that and so dark data is this concept of you know what? we don't you know you don't know what you don't know and therefore there's so much data that you have that. Over half the day that you own you don't even know how to look at it and that's starting to change with Ai you know the tools that ais allows can help kind of change that and but we still a lot of work to do even on what we do know to get the insights out of there first. So there's a lot more lot more work to be done.
31:58.10
mike_flywheel
For got it. So for companies thinking about this. It's like there's people that are hey I don't know what I don't know and I just want to reason over some data hypothetically but you're seeing a lot of people that are like no, we're we're reasoning over the data today. We're analyzing it. We're reporting on it. We're trying to snap Ai to it.
32:06.14
Josh Gray
Um, yeah, yeah.
32:16.18
mike_flywheel
We know what we want to do we are doing it. It's just super inefficient. You come in to solve that efficiency and so how do you how do you charge for this how does that work is it you know throughput based monthly subscription base how how do you charge for Artemis so
32:19.94
Josh Gray
Yeah.
32:28.69
Josh Gray
Yeah, it's good question I mean we're an early startup So we're always experimenting but it that way. Yeah, um, so right now you know we're really trying to understand more about our user and understand how they want to use the platform Currently, we've done a per c.
32:33.86
mike_flywheel
It's free. Yeah.
32:43.63
Josh Gray
A month basis. But we probably you know we've looked at changing to or more usage basis in the data industry though you have to be somewhat careful with usage just because there's been a bit of abuse I'd say with data platforms. You know, claiming up the uses base and then you know you get it built in a month you're like oh my gosh like why is it. You know a million dollars instead of. Ah, half a million that I thought it was going to be so I'll say we're still experimenting with a lot of that stuff. But right now it's it's purely just ah, a user per month Sas fee.
33:12.38
mike_flywheel
That's cool so where where are you at in the journey. How big's the team. How many customers sort of what's the year head look like give us ah, give us the snapshot.
33:18.62
Josh Gray
Yeah, so we've been. We've been in business actually about two years next week so it's it's been pretty crazy wild journey that we're you know, thankful that we're so alive. Um, we're 6 people strong so we actually just expanded our team which has been amazing and we got our first office this year we're based out here in Vancouver canada so we love that. Um, and yeah, we're we've just actually raised ah our kind of presc round so to speak from amazing investors. So that's that's really awesome from there and from a customer side. We're working with about 50 organizations just over. Um, you know couple hundred active users which has been great. So we're getting a lot of good feedback understanding really what these customer workflows are because you know we want this product to be you know a way for analysts to have you know, sleep better at night and so the more we can reach the better.
34:06.83
mike_flywheel
You got it and if people want to find out more sign up. Is it just through your website. We'll have everything in the description but where should people people know.
34:11.48
Josh Gray
Yeah, yeah, yeah, check out, check out our website you know, connect with me on Linkedin I'm always happy to talk to people you know I know the founder journey can be. You know can be a struggle and so I'm always you know keep my keep my doors open for that if people want to talk about you know building companies want to talk about data.
34:28.92
mike_flywheel
I got it. That's artemmisdata Io Correct Awesome now. The other thing I Always like to ask for anyone that comes on because you never know who our listeners are going to be but is there anything like in your Artemmis journey other than people signing up if they're interested to learn more.
34:29.27
Josh Gray
I'm always happy to do So yeah.
34:46.50
mike_flywheel
That would be helpful. Anybody you're looking to connect with anything that would help accelerate what you're doing so.
34:50.00
Josh Gray
Yeah I mean we're always looking for data people. So If you're a data analyst or you run a data team or you're an and Ml engineer or whatever you know, come come say hi we you know we love talking to people in the data world understanding their problems. You know. Regardless of if we're going to work with you or not yeah, we always want to meet people like that. So.
35:09.94
mike_flywheel
So that's cool. Well and I have to ask you've had 1 successful exit. You're kicking ass and you seem to have cut the right you know headwinds to capture this ai you know fire sale of what's happening right now. Um, what like what.
35:23.80
Josh Gray
Um, yeah.
35:26.30
mike_flywheel
Has been the most useful advice that you've sort of embraced on either this journey or the previous one. Maybe it's been both I find it's always like something that's stuck with you from from others that maybe you think others should hear.
35:36.30
Josh Gray
Yeah I think that's a good question I think really just solving 1 problem at a time. This stuff is a lot more complicated than it looks you know there you know I think there's ah, there's an interview with Jensen one of the founders. The.
35:45.69
mike_flywheel
So okay.
35:55.75
Josh Gray
Nvidia and he was like you know if I to do all over again. I wouldn't because it's you know it's brutal this truth to that I think you know I was very lucky early on in my entrepreneurial journey that I had a win that I could kind of build off but building a business is difficult. There's a lot of challenges a lot of ups and downs. But if you just focus one day at a time 1 problem at a time. These problems aren't you know insurmountable. It's just a matter of solving one after the other and so you know for anyone out there I'd say just keep on solving them live to fight another day and you should be getting the long run.
36:23.71
mike_flywheel
I I like it I think someone wrapped that up 1 time and and called it like just make sure you focus on putting one foot in front of the other and then you will end up walking yourself tens of thousands of kilometers so focus on the one foot in front of the other. Okay.
36:32.10
Josh Gray
Hundred percent yeah Yeah it's ah it's amazing. You know, looking to to you know back for the last two years never in 1000000 years would I have ever imagined the people I would have not gotten to know the investors that we have on board the partners the customers people that we were able to talk to I could have dreamed it up if I wanted to um, but yet it just comes by putting one foot in front of the other and you know solving those problems so keep at it.
36:59.60
mike_flywheel
What's what's you know, along that has there been any like tools or um approaches that you think have been like useful to you or that you and the team are using now I think like.
37:09.12
Josh Gray
Yeah, yeah.
37:10.57
mike_flywheel
You know I think I'm gonna be saying Chad Gp because I want to hear like what are like tools or approaches other people are using that that might be useful for other founders starting their journey and.
37:20.28
Josh Gray
I mean if I know y comminary if you go to their Youtube channel they have a ton of awesome videos. They have such a good library and they basically open source like how to build a company. Um you know I go there for a lot of stuff just learning understanding. You know, different perspectives and different ideas I think sometimes when you're a small team. Maybe you're the only person on the business side. Maybe ah, you're the only engineer you can kind of forget to be challenged and you forget to you know you forget to remember there's other ideas in the world and you know your idea isn't necessarily the best way to do something and so I always try to bounce off other things understand. Okay, here's how I think. How does the world see it what's it maybe another perspective that will open up by how I look at things but having that openness is going to is going to save you a lot of headache in in the future.
38:00.35
mike_flywheel
Now on the flip side is there anything that having gone through the exit of one company. The build of another anything that like maybe you looked over in one of those elements of the journey that you're like hey if I went back here's how I would modify my approach. You know you even talked about that exit of that first company and you know it's a lot longer and a little bit more complicated than people expect is there anything you would change how you set up the company something that's like a watch that maybe people don't catch early enough.
38:19.52
Josh Gray
And yet.
38:30.25
Josh Gray
I mean I wouldn't say I've been lucky with this but I would say just make sure you that you you're aware of your team. You know I'm very fortunate that I work with amazing people and I love working with them in both the business I've had um but I know lots of founders who haven't and they've had issues and and those issues are honestly.
38:36.90
mike_flywheel
And.
38:50.70
Josh Gray
Really what kill companies and so do not overlook the team do not underestimate how powerful taking the right team can be um, it literally is the difference between being successful or not.
38:59.83
mike_flywheel
Any tips on that like is there something that you know in your first couple hires that you found to be a critical thing that you look for or hire for that worked out. Well even though maybe you didn't intentionally think about it I'm an end.
39:09.67
Josh Gray
I Mean this is like not going to help. Yeah I mean this probably a help but I mean trust your good I think people I think as people were very aware of other people. The minute you kind of meet them and you kind of you kind of get that feeling when you meet somebody Obviously that could be wrong and then know those times when that happened But. Trust your gut when it comes around people. You're normally right and so just trust it and and make those decisions quickly because yeah, they kind of a huge impact.
39:32.84
mike_flywheel
Yeah, what? what's that look like for you is it like hey you want to meet the person. Virtually let's go over for our coffee or a beer together I Want to understand you who you are or you able to judge that pretty quickly just over a virtual call is they're like just curious. How.
39:43.47
Josh Gray
Um, yeah I think pretty quickly I think I mean I think we're all people we all know what when somebody's being genuine and when someone's not being genuine. Um, so just trust that I think it's really easy to you know? Maybe someone's coming in with great credentials and.
39:53.60
mike_flywheel
So yeah, so.
40:01.70
Josh Gray
You're you're shocked at why they'd even consider working with you Just always be aware. Yeah exactly? Yeah yeah.
40:07.36
mike_flywheel
Bittcon for anyone that has much bitcon. Yeah, you just put you know, put Harvard on your Linkedin. It's all good. Well you know Josh it's been a ah pleasure talking to you today I think what you're solving is very much needed Artemis automating data cleanup with with ai. Um, I'm excited to watch your journey and follow along see if there's ways I can help thanks for coming on the show today. Any closing words on your side for for our audience Thanks.
40:31.67
Josh Gray
Yeah, hope you enjoyed the the content if you have any questions feel free to reach out.
40:36.45
mike_flywheel
I love it. Thanks everyone who tuned into the pitch please podcast again. That's Josh with artemmis automating data cleanup with Ai artemisdata dot io make sure to check them out details in the description. We'll see you all on the next episode. Thanks again. Thanks.
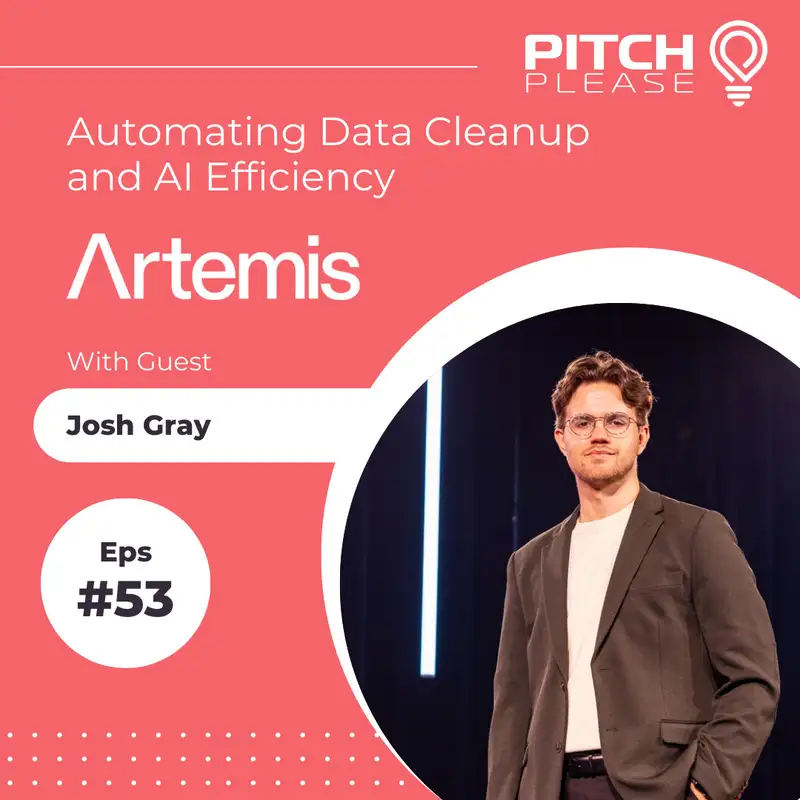